Optimize the calculation of horizontal and vertical adjacency using numpy(使用numpy优化水平和垂直邻接计算)
本文介绍了使用numpy优化水平和垂直邻接计算的处理方法,对大家解决问题具有一定的参考价值,需要的朋友们下面随着小编来一起学习吧!
问题描述
我有以下单元格:
cells = np.array([[1, 1, 1],
[1, 1, 0],
[1, 0, 0],
[1, 0, 1],
[1, 0, 0],
[1, 1, 1]])
我要计算水平邻接和垂直邻接以得出此结果:
# horizontal adjacency
array([[3, 2, 1],
[2, 1, 0],
[1, 0, 0],
[1, 0, 1],
[1, 0, 0],
[3, 2, 1]])
# vertical adjacency
array([[6, 2, 1],
[5, 1, 0],
[4, 0, 0],
[3, 0, 1],
[2, 0, 0],
[1, 1, 1]])
实际解决方案如下:
def get_horizontal_adjacency(cells):
adjacency_horizontal = np.zeros(cells.shape, dtype=int)
for y in range(cells.shape[0]):
span = 0
for x in reversed(range(cells.shape[1])):
if cells[y, x] > 0:
span += 1
else:
span = 0
adjacency_horizontal[y, x] = span
return adjacency_horizontal
def get_vertical_adjacency(cells):
adjacency_vertical = np.zeros(cells.shape, dtype=int)
for x in range(cells.shape[1]):
span = 0
for y in reversed(range(cells.shape[0])):
if cells[y, x] > 0:
span += 1
else:
span = 0
adjacency_vertical[y, x] = span
return adjacency_vertical
算法基本为(水平邻接):
- 行间循环
- 跨列向后循环
- 如果单元格的x,y值不是零,则在实际跨度上加1
- 如果单元格的x,y值为零,则将实际跨度重置为零
- 将跨度设置为结果数组的新x,y值
因为我需要在所有数组元素上循环两次,所以对于较大的数组(例如图像),这会很慢。
有没有办法使用矢量化或其他一些数字魔术来改进算法?
摘要:
Joni和Mark Setchell提出了很棒的建议!
我使用示例图像和带有比较的python文件创建了一个small Repo。结果令人震惊:
- 原方案:3.675秒
- 使用Numba:0.002 s
- 使用Cython:0.005 s
推荐答案
我用Numba快速尝试了一下,但没有检查得太彻底,尽管结果似乎大致正确:
#!/usr/bin/env python3
# https://stackoverflow.com/q/69854335/2836621
# magick -size 1920x1080 xc:black -fill white -draw "circle 960,540 960,1040" -fill black -draw "circle 960,540 960,800" a.png
import cv2
import numpy as np
import numba as nb
def get_horizontal_adjacency(cells):
adjacency_horizontal = np.zeros(cells.shape, dtype=int)
for y in range(cells.shape[0]):
span = 0
for x in reversed(range(cells.shape[1])):
if cells[y, x] > 0:
span += 1
else:
span = 0
adjacency_horizontal[y, x] = span
return adjacency_horizontal
@nb.jit('void(uint8[:,::1], int32[:,::1])',parallel=True)
def nb_get_horizontal_adjacency(cells, result):
for y in nb.prange(cells.shape[0]):
span = 0
for x in range(cells.shape[1]-1,-1,-1):
if cells[y, x] > 0:
span += 1
else:
span = 0
result[y, x] = span
return
# Load image
im = cv2.imread('a.png', cv2.IMREAD_GRAYSCALE)
%timeit get_horizontal_adjacency(im)
result = np.zeros((im.shape[0],im.shape[1]),dtype=np.int32)
%timeit nb_get_horizontal_adjacency(im, result)
计时很好,如果工作正常,显示4000倍的加速:
In [15]: %timeit nb_get_horizontal_adjacency(im, result)
695 µs ± 9.12 µs per loop (mean ± std. dev. of 7 runs, 1000 loops each)
In [17]: %timeit get_horizontal_adjacency(im)
2.78 s ± 44.2 ms per loop (mean ± std. dev. of 7 runs, 1 loop each)
输入
使用ImageMagick创建1080p尺寸(即1920x1080)的输入图像,使用:
magick -size 1920x1080 xc:black -fill white -draw "circle 960,540 960,1040" -fill black -draw "circle 960,540 960,800" a.png
输出(对比度调整)
这篇关于使用numpy优化水平和垂直邻接计算的文章就介绍到这了,希望我们推荐的答案对大家有所帮助,也希望大家多多支持编程学习网!
沃梦达教程
本文标题为:使用numpy优化水平和垂直邻接计算
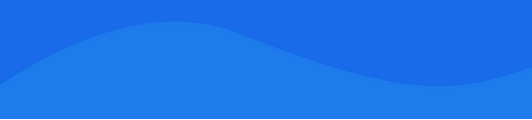
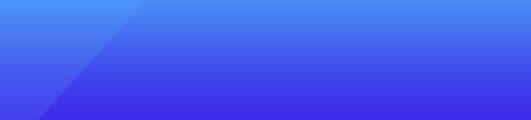
猜你喜欢
- 使用Heroku上托管的Selenium登录Instagram时,找不到元素';用户名'; 2022-01-01
- python-m http.server 443--使用SSL? 2022-01-01
- 如何将一个类的函数分成多个文件? 2022-01-01
- 分析异常:路径不存在:dbfs:/databricks/python/lib/python3.7/site-packages/sampleFolder/data; 2022-01-01
- 如何在 Python 的元组列表中对每个元组中的第一个值求和? 2022-01-01
- pytorch 中的自适应池是如何工作的? 2022-07-12
- 如何在 python3 中将 OrderedDict 转换为常规字典 2022-01-01
- python check_output 失败,退出状态为 1,但 Popen 适用于相同的命令 2022-01-01
- padding='same' 转换为 PyTorch padding=# 2022-01-01
- 沿轴计算直方图 2022-01-01