How to implement Matlab#39;s mldivide (a.k.a. the backslash operator quot;quot;)(如何实现 Matlab 的 mldivide(又名反斜杠运算符“))
问题描述
我目前正在尝试开发一个面向矩阵的小型数学库(我正在使用 中都有对应的方法,实际上这可能是MATLAB正在做的(请注意,最新版本的 MATLAB 附带优化的 Intel MKL 实现).
采用不同方法的原因是它试图使用最具体的算法来求解利用系数矩阵所有特征的方程组(或者因为它会更快或更数值稳定).所以你当然可以使用通用求解器,但它不会是最有效的.
事实上,如果您事先知道 A
是什么样的,您可以通过调用 linsolve
并直接指定选项.
如果 A
是矩形或单数,你也可以使用 PINV 找到最小范数最小二乘解(使用 SVD 分解):
x = pinv(A)*b
以上所有内容都适用于稠密矩阵,稀疏矩阵则完全不同.通常迭代求解器用于此类案件.我相信 MATLAB 使用 UMFPACK 和 SuiteSpase 包中的其他相关库用于直接求解器.
在处理稀疏矩阵时,您可以打开诊断信息并查看使用 sparms
:
sparms('spumoni',2)x = A;
此外,反斜杠运算符也适用于 gpuArray,在在这种情况下,它依赖于 cuBLAS 和 MAGMA 在 GPU 上执行.
它也适用于 分布式数组,适用于分布式计算环境(工作在一组计算机中分配,其中每个工作人员只有数组的一部分,可能整个矩阵不能一次全部存储在内存中).底层实现使用 ScaLAPACK.
如果你想自己实现所有这些,那是一个非常艰巨的任务:)
I'm currently trying to develop a small matrix-oriented math library (I'm using Eigen 3 for matrix data structures and operations) and I wanted to implement some handy Matlab functions, such as the widely used backslash operator (which is equivalent to mldivide ) in order to compute the solution of linear systems (expressed in matrix form).
Is there any good detailed explanation on how this could be achieved ? (I've already implemented the Moore-Penrose pseudoinverse pinv function with a classical SVD decomposition, but I've read somewhere that A
isn't always pinv(A)*b
, at least Matalb doesn't simply do that)
Thanks
For x = A
, the backslash operator encompasses a number of algorithms to handle different kinds of input matrices. So the matrix A
is diagnosed and an execution path is selected according to its characteristics.
The following page describes in pseudo-code when A
is a full matrix:
if size(A,1) == size(A,2) % A is square
if isequal(A,tril(A)) % A is lower triangular
x = A b; % This is a simple forward substitution on b
elseif isequal(A,triu(A)) % A is upper triangular
x = A b; % This is a simple backward substitution on b
else
if isequal(A,A') % A is symmetric
[R,p] = chol(A);
if (p == 0) % A is symmetric positive definite
x = R (R' b); % a forward and a backward substitution
return
end
end
[L,U,P] = lu(A); % general, square A
x = U (L (P*b)); % a forward and a backward substitution
end
else % A is rectangular
[Q,R] = qr(A);
x = R (Q' * b);
end
For non-square matrices, QR decomposition is used. For square triangular matrices, it performs a simple forward/backward substitution. For square symmetric positive-definite matrices, Cholesky decomposition is used. Otherwise LU decomposition is used for general square matrices.
Update: MathWorks has updated the algorithm section in the doc page of
mldivide
with some nice flow charts. See here and here (full and sparse cases).
All of these algorithms have corresponding methods in LAPACK, and in fact it's probably what MATLAB is doing (note that recent versions of MATLAB ship with the optimized Intel MKL implementation).
The reason for having different methods is that it tries to use the most specific algorithm to solve the system of equations that takes advantage of all the characteristics of the coefficient matrix (either because it would be faster or more numerically stable). So you could certainly use a general solver, but it wont be the most efficient.
In fact if you know what A
is like beforehand, you could skip the extra testing process by calling linsolve
and specifying the options directly.
if A
is rectangular or singular, you could also use PINV to find a minimal norm least-squares solution (implemented using SVD decomposition):
x = pinv(A)*b
All of the above applies to dense matrices, sparse matrices are a whole different story. Usually iterative solvers are used in such cases. I believe MATLAB uses UMFPACK and other related libraries from the SuiteSpase package for direct solvers.
When working with sparse matrices, you can turn on diagnostic information and see the tests performed and algorithms chosen using spparms
:
spparms('spumoni',2)
x = A;
What's more, the backslash operator also works on gpuArray's, in which case it relies on cuBLAS and MAGMA to execute on the GPU.
It is also implemented for distributed arrays which works in a distributed computing environment (work divided among a cluster of computers where each worker has only part of the array, possibly where the entire matrix cannot be stored in memory all at once). The underlying implementation is using ScaLAPACK.
That's a pretty tall order if you want to implement all of that yourself :)
这篇关于如何实现 Matlab 的 mldivide(又名反斜杠运算符“")的文章就介绍到这了,希望我们推荐的答案对大家有所帮助,也希望大家多多支持编程学习网!
本文标题为:如何实现 Matlab 的 mldivide(又名反斜杠运算符“&
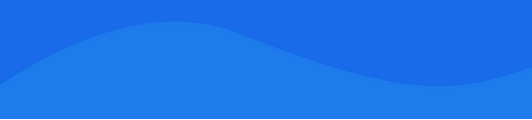
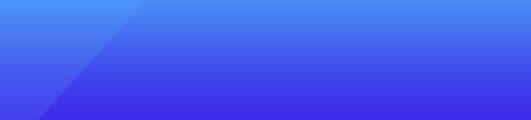
- DoEvents 等效于 C++? 2021-01-01
- 从父 CMakeLists.txt 覆盖 CMake 中的默认选项(...)值 2021-01-01
- 如何提取 __VA_ARGS__? 2022-01-01
- OpenGL 对象的 RAII 包装器 2021-01-01
- 使用 __stdcall & 调用 DLLVS2013 中的 GetProcAddress() 2021-01-01
- GDB 不显示函数名 2022-01-01
- 将 hdc 内容复制到位图 2022-09-04
- XML Schema 到 C++ 类 2022-01-01
- 哪个更快:if (bool) 或 if(int)? 2022-01-01
- 将函数的返回值分配给引用 C++? 2022-01-01